Archive
Software research is 200 years behind biology research
Evidence-based software research requires access to data, and Github has become the primary source of raw material for many (most?) researchers.
Parallels are starting to emerge between today’s researchers exploring Github and biologists exploring nature centuries ago.
Centuries ago scientific expeditions undertook difficult and hazardous journeys to various parts of the world, collecting and returning with many specimens which were housed and displayed in museums and botanical gardens. Researchers could then visit the museums and botanical gardens to study these specimens, without leaving the comforts of their home country. What is missing from these studies of collected specimens is information on the habitat in which they lived.
Github is a living museum of specimens that today’s researchers can study without leaving the comforts of their research environment. What is missing from these studies of collected specimens is information on the habitat in which the software was created.
Github researchers are starting the process of identifying and classifying specimens into species types, based on their defining characteristics, much like the botanist Carl_Linnaeus identified stamens as one of the defining characteristics of flowering plants. Some of the published work reads like the authors did some measurements, spotted some differences, and then invented a plausible story around what they had found. As a sometime inhabitant of this glasshouse I will refrain from throwing stones.
Zoologists study the animal kingdom, and entomologists specialize in the insect world, e.g., studying Butterflys. What name might be given to researchers who study software source code, and will there be specialists, e.g., those who study cryptocurrency projects?
The ecological definition of a biome, as the community of plants and animals that have common characteristics for the environment they exist in, maps to the end-user use of software systems. There does not appear to be a generic name for people who study the growth of plants and animals (or at least I cannot think of one).
There is only so much useful information that can be learned from studying specimens in museums, no matter how up to date the specimens are.
Studying the development and maintenance of software systems in the wild (i.e., dealing with the people who do it), requires researchers to forsake their creature comforts and undertake difficult and hazardous journeys into industry. While they are unlikely to experience any physical harm, there is a real risk that their egos will be seriously bruised.
I want to do what I can to prevent evidence-based software engineering from just being about mining Github. So I have a new policy for dealing with PhD/MSc student email requests for data (previously I did my best to point them at the data they sought). From now on, I will tell students that they need to behave like real researchers (e.g., Charles Darwin) who study software development in the wild. Charles Darwin is a great role model who should appeal to their sense of adventure (alternative suggestions welcome).
What software engineering data have I collected on subject X?
While it’s great that so much data was uncovered during the writing of the Evidence-based software engineering book, trying to locate data on a particular topic can be convoluted (not least because there might not be any). There are three sources of information about the data:
- the paper(s) written by the researchers who collected the data,
- my analysis and/or discussion of the data (which is frequently different from the original researchers),
- the column names in the csv file, i.e., data is often available which neither the researchers nor I discuss.
At the beginning I expected there to be at most a few hundred datasets; easy enough to remember what they are about. While searching for some data, one day, I realised that relying on memory was not a good idea (it was never a good idea), and started including data identification tags in every R file (of which there are currently 980+). This week has been spent improving tag consistency and generally tidying them up.
How might data identification information be extracted from the paper that was the original source of the data (other than reading the paper)?
Named-entity recognition, NER, is a possible starting point; after all, the data has names associated with it.
Tools are available for extracting text from pdf file, and 10-lines of Python later we have a list of named entities:
import spacy # Load English tokenizer, tagger, parser, NER and word vectors nlp = spacy.load("en_core_web_sm") file_name = 'eseur.txt' soft_eng_text = open(file_name).read() soft_eng_doc = nlp(soft_eng_text) for ent in soft_eng_doc.ents: print(ent.text, ent.start_char, ent.end_char, ent.label_, spacy.explain(ent.label_)) |
The catch is that en_core_web_sm is a general model for English, and is not software engineering specific, i.e., the returned named entities are not that good (from a software perspective).
An application domain language model is likely to perform much better than a general English model. While there are some application domain models available for spaCy (e.g., biochemistry), and application datasets, I could not find any spaCy models for software engineering (I did find an interesting word2vec model trained on Stackoverflow posts, which would be great for comparing documents, but not what I was after).
While it’s easy to train a spaCy NER model, the time-consuming bit is collecting and cleaning the text needed. I have plenty of other things to keep me busy. But this would be a great project for somebody wanting to learn spaCy and natural language processing 🙂
What information is contained in the undiscussed data columns? Or, from the practical point of view, what information can be extracted from these columns without too much effort?
The number of columns in a csv file is an indicator of the number of different kinds of information that might be present. If a csv is used in the analysis of X, and it contains lots of columns (say more than half-a-dozen), then it might be assumed that it contains more data relating to X.
Column names are not always suggestive of the information they contain, but might be of some use.
Many of the csv files contain just a few rows/columns. A list of csv files that contain lots of data would narrow down the search, at least for those looking for lots of data.
Another possibility is to group csv files by potential use of data, e.g., estimating, benchmarking, testing, etc.
More data is going to become available, and grouping by potential use has the advantage that it is easier to track the availability of new data that may supersede older data (that may contain few entries or apply to circumstances that no longer exist)
My current techniques for locating data on a given subject is either remembering the shape of a particular plot (and trying to find it), or using the pdf reader’s search function to locate likely words and phrases (and then look at the plots and citations).
Suggestions for searching or labelling the data, that don’t require lots of effort, welcome.
Researching programming languages
What useful things might be learned from evidence-based research into programming languages?
A common answer is researching how to design a programming language having a collection of desirable characteristics; with desirable characteristics including one or more of: supporting the creation of reliable, maintainable, readable, code, or being easy to learn, or easy to understand, etc.
Building a theory of, say, code readability is an iterative process. A theory is proposed, experiments are run, results are analysed; rinse and repeat until a theory having a good enough match to human behavior is found. One iteration will take many years: once a theory is proposed, an implementation has to be built, developers have to learn it, and spend lots of time using it to enable longer term readability data to be obtained. This iterative process is likely to take many decades.
Running one iteration will require 100+ developers using the language over several years. Why 100+? Lots of subjects are needed to obtain statistically meaningful results, people differ in their characteristics and previous software experience, and some will drop out of the experiment. Just one iteration is going to cost a lot of money.
If researchers do succeed in being funded and eventually discovering some good enough theories, will there be a mass migration of developers to using languages based on the results of the research findings? The huge investment in existing languages (both in terms of existing code and developer know-how) means that to stand any chance of being widely adopted these new language(s) are going to have to deliver a substantial benefit.
I don’t see a high cost multi-decade research project being funded, and based on the performance improvements seen in studies of programming constructs I don’t see the benefits being that great (benefits in use of particular constructs may be large, but I don’t see an overall factor of two improvement).
I think that creating new programming languages will continue to be a popular activity (it is vanity research), and I’m sure that the creators of these languages will continue to claim that their language has some collection of desirable characteristics without any evidence.
What programming research might be useful and practical to do?
One potentially practical and useful question is the lifecycle of programming languages. Where the components of the lifecycle includes developers who can code in the language, source code written in the language, and companies dependent on programs written in the language (who are therefore interested in hiring people fluent in the language).
Many languages come and go without many people noticing, a few become popular for a few years, and a handful continue to be widely used over decades. What are the stages of life for a programming language, what factors have the largest influence on how widely a language is used, and for how long it continues to be used?
Sixty years worth of data is waiting to be collected and collated; enough to keep researchers busy for many years.
The uses of a lifecycle model, that I can thinkk of, all involve the future of a language, e.g., how much of a future does it have and how might it be extended.
Some recent work looking at the rate of adoption of new language features includes: On the adoption, usage and evolution of Kotlin Features on Android development, and Understanding the use of lambda expressions in Java; also see section 7.3.1 of Evidence-based software engineering.
Evidence-based software engineering: book released
My book, Evidence-based software engineering, is now available; the pdf can be downloaded here, here and here, plus all the code+data. Report any issues here. I’m investigating the possibility of a printed version. Mobile friendly pdf (layout shaky in places).
The original goals of the book, from 10-years ago, have been met, i.e., discuss what is currently known about software engineering based on an analysis of all the publicly available software engineering data, and having the pdf+data+code freely available for download. The definition of “all the public data” started out as being “all”, but as larger and higher quality data was discovered the corresponding were ignored.
The intended audience has always been software developers and their managers. Some experience of building software systems is assumed.
How much data is there? The data directory contains 1,142 csv files and 985 R files, the book cites 895 papers that have data available of which 556 are cited in figure captions; there are 628 figures. I am currently quoting the figure of 600+ for the ‘amount of data’.
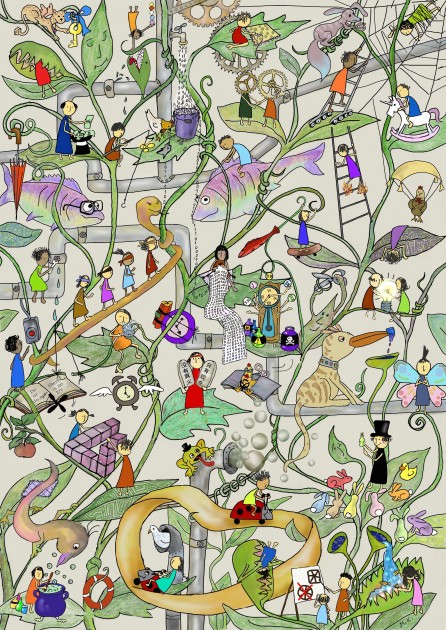
Things that might be learned from the analysis has been discussed in previous posts on the chapters: Human cognition, Cognitive capitalism, Ecosystems, Projects and Reliability.
The analysis of the available data is like a join-the-dots puzzle, except that the 600+ dots are not numbered, some of them are actually specs of dust, and many dots are likely to be missing. The future of software engineering research is joining the dots to build an understanding of the processes involved in building and maintaining software systems; work is also needed to replicate some of the dots to confirm that they are not specs of dust, and to discover missing dots.
Some missing dots are very important. For instance, there is almost no data on software use, but there can be lots of data on fault experiences. Without software usage data it is not possible to estimate whether the software is very reliable (i.e., few faults experienced per amount of use), or very unreliable (i.e., many faults experienced per amount of use).
The book treats the creation of software systems as an economically motivated cognitive activity occurring within one or more ecosystems. Algorithms are now commodities and are not discussed. The labour of the cognitariate is the means of production of software systems, and this is the focus of the discussion.
Existing books treat the creation of software as a craft activity, with developers applying the skills and know-how acquired through personal practical experience. The craft approach has survived because building software systems has been a sellers market, customers have paid what it takes because the potential benefits have been so much greater than the costs.
Is software development shifting from being a sellers market to a buyers market? In a competitive market for development work and staff, paying people to learn from mistakes that have already been made by many others is an unaffordable luxury; an engineering approach, derived from evidence, is a lot more cost-effective than craft development.
As always, if you know of any interesting software engineering data, please let me know.
The Weirdest people in the world
Western, Educated, Industrialized, Rich and Democratic: WEIRD people are the subject of Joseph Henrich’s latest book “The Weirdest People in the World: How the West Became Psychologically Peculiar and Particularly Prosperous”.
This book is in the mold of Jared Diamond’s Guns, Germs, and Steel: The Fates of Human Societies, but comes at the topic from a psychological/sociological angle.
This very readable book is essential reading for anyone wanting to understand how very different WEIRD people are, along with the societies they have created, compared to people and societies in the rest of the world today and the entire world up until around 500 years ago.
The analysis of WEIRD people/societies has three components: why we are different (I’m assuming that most of this blog’s readers are WEIRD), the important differences that are known about, and the cultural/societal consequences (the particularly prosperous in the subtitle is a big clue).
Henrich cites data to back up his theories.
Starting around 1,500 years ago the Catholic church started enforcing a ban on cousin marriage, which was an almost universal practice at the time and is still widely practiced in non-WEIRD societies. Over time the rules got stricter, until by the 11th century people were not allowed to marry anyone related out to their sixth cousin. The rules were not always strictly enforced, as Henrich documents, but the effect was to change the organization of society from being kin-based to being institution-based (in particular institutions such as the Church and state). Finding a wife/husband required people to interact with others outside their extended family.
Effects claimed, operating over centuries, of the shift from extended families to nuclear families are that people learned what Henrich calls “impersonal prosociality”, e.g., feeling comfortable dealing with strangers. People became more altruistic, the impartial rule of law spread (including democracy and human rights), plus other behaviors needed for the smooth running of large social units (such as towns, cities and countries).
The overall impact was that social units of WEIRD people could grow to include tens of thousands, even millions, or people, and successfully operate at this scale. Information about beneficial inventions could diffuse rapidly and people were free(ish) to try out new things (i.e., they were not held back by family customs), and operating in a society with free movement of people there were lots of efficiencies, e.g., companies were not obligated to hire family members, and could hire the best person they could find.
Consequently, the West got to take full advantage of scientific progress, invent and mass produce stuff. Outcompeting the non-WEIRD world.
The big ideas kind of hang together. Some of the details seem like a bit of a stretch, but I’m no expert.
My WEIRD story occurred about five years ago, when I was looking for a publisher for the book I was working on. One interested editor sent out an early draft for review. One of the chapters discusses human cognition, and I pointed out that it did not matter that most psychology experiments had been done using WEIRD subjects, because software developers were WEIRD (citing Henrich’s 2010 WEIRD paper). This discussion of WEIRD people was just too much for one of the reviewers, who sounded like he was foaming at the mouth when reviewing my draft (I also said a few things about academic researchers that upset him).
Recent Comments