Archive
Descriptive statistics of some Agile feature characteristics
The purpose of software engineering research is to figure out how software development works so that the software industry can improve its quality/timeliness (i.e., lower costs and improved customer satisfaction). Research is hampered by the fact that companies are not usually willing to make public good quality data about the details of their software development processes.
In mid July a post on the ACCU general mailing list caught my eye and I followed a link to a very interesting report, went to visit 7digital a few weeks later, told them about my empirical software engineering with R book and how I wanted to make all the data I used available to readers and they agreed to make the data public! The data arrived at the start of August and I spent the rest of the month analyzing it (the R code I used to analyse it).
Below is a draft of what will eventually appear in the book. As always comments welcome, particularly if you can extract more information from the 7digital data (the mapping of material to WordPress blog format might still be flaky in places).
Agile feature characteristics
Traditionally software development projects work towards releasing product updates on prespecified dates, often with a release cycle of between once or twice a year and with many updates included in each release. In contrast to this approach development groups following an Agile method <book ???> make frequent releases with each containing a small incremental update (Agile is an umbrella term applied to a variety of iterative and incremental software development methodologies).
Rationale for the Agile approach includes getting rapid feedback from customers on the direction of developments and maximizing return on software investment by getting newly implemented features into customers hand almost immediately.
The large number of releases (compared to other approaches) has the potential to provide enough data for meaningful statistical analysis of questions such as how often new features are released and the number of features under development at any time.
7digital<book 7Digital_12> is a digital media delivery company that operates an international on-line digital music store (www.7digital.com) and provides business to business digital media services via an open API platform. At 7digital software development is done using an Agile process and since April 2009 various items of information have been recorded <book Bowley_12>; 7digital are open about there process and have made this information publicly available and it is analysed here.
Data
The data consists of information on the 3,238 features implemented by the 7digital team between April 2009 and July 2012; this information consists of three dates (Prioritised/Start Development/Done), a classification of the feature as one of nine possible internal types (i.e.,
During the recording period the number of developers grew from 14 to 35.
The start/done dates represent an elapsed time period, a wide variety of factors can cause work on the implementation of a feature to be stalled for a period of time, i.e., the time difference need not represent total development time.
The Agile process gives a great deal of flexibility to developers about which projects they chose to work on. Information on the number of developers working on the implementation of individual features was not recorded.
Is the data believable?
As discussed elsewhere [checking data quality] measurements involving people are likely to be subject more external influences than measurements of inanimate objects such as source code, they are also more difficult to replicate and are open to those being measured influencing the results in their favor.
The following is what is known about the 7digital measurement process.
The data recording was done by whoever ran the Agile stand-up session at the start of the day.
What unit of time measurement is appropriate for analysing an Agile process? While fine grained measurements are the ideal they have the potential to require nontrivial effort from those reporting the values, are open to individual interpretation (e.g., when exactly did work start/stop on this feature?) and subject to human error (e.g., forgetting to note the event when it happened and having to recall it later). The day was chosen as the basic unit of time measurement; in light of the time needed to implement most features this may seem too large, but this choice has the advantage of being the natural unit of measurement in that developers meet together every morning to discuss progress and that days work and being so broad makes it more likely that start/end times will be consistently applied as well as less prone to inaccurate recall later.
Goodhart’s law (it is really an observation of human behavior rather than a law) says “Any observed statistical regularity will tend to collapse once pressure is placed on it for control purposes.” If the measurements collected were actively used to control or evaluate the development team then the developers would be motivated to move the measurements in the direction that was favorable to them. 7digital do not attempt to use the measurements for control or evaluation or developers and developers have no motive change their behavior based on being measured.
I find the data believable in that the measurement process is not so expensive or cumbersome that developers are unwilling to attempt to report accurate data and not being directly effected by the results means they have no motive for changing their behavior to influence the measurements.
Believable data does not mean the data is error free. The following is a count of the days of the week on which feature implementations were recorded as being Done. Monday is day 0 and the counts for Saturday/Sunday should be zero; assuming that Friday/Monday had been intended the non-zero values suggest a 2-4% error rate, comparable with human error rates for low stress/non-critical work.
> table(Done.day[(Done.day <= 650)] %% 7) 0 1 2 3 4 5 6 227 225 214 243 177 8 7 > table(Done.day[(Done.day > 650)] %% 7) 0 1 2 3 4 5 6 443 483 455 473 270 4 9 |
Predictions made in advance
Your author is not aware of any empirically based theory of Agile feature development capable of making predictions about development time related questions.
The analysis described here is purely descriptive; there is no attempt to build predictive models or compare the data against any existing theory.
The results from this data analysis (and all analysis in this book) are to provide information that will help software developers do a better job. What information can be extracted that would be useful to 7digital? This has proved to be a something of a chicken-and-egg question because people are interested in seeing the results before deciding whether they are useful. The following issues are of general interest:
- characteristics of the time taken to implement new features,
- variations in the number of different kinds of features (e.g., bug/non-bug) over time,
Applicable techniques
Overview of data
The data consists of start/finish times for the implementations of features and the overview information that springs to mind is average number of features implementation starts per time interval and average time taken to implement a feature. The figure below is a good enough approximation to this information to get a rough idea of its characteristics (e.g., the effect of weekends and holidays have not been taken into account and a 30 day rolling mean has been applied to smooth out daily fluctuations).
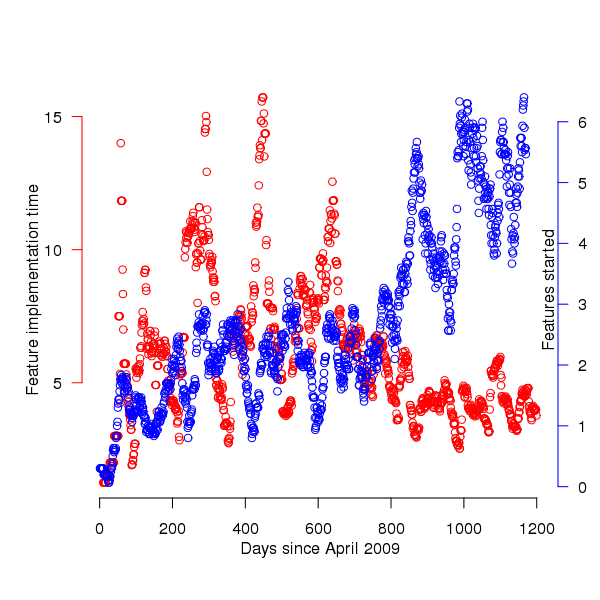
Figure 1. Average number of feature implementations started (blue) and their average duration (red); a 30 day rolling mean has been applied to both. Data courtesy of 7digital.
The plot appears to have two parts, before and after day 650 (or thereabouts). After day 650 the oscillations in feature implementation time die down substantially and the rate at which new feature implementations are started steadily increases. Possible reasons for the larger variations in the first 650 days include less expertise in organizing features into smaller work items and larger features being needed during the earlier stages of product development.
Obviously shorter implementation times make it possible to start work on more new features, however new feature starts continues to increase while implementation time stabilises around a lower value. Possible causes for the continuing increase in new feature starts include an increase in the number of developers and/or existing developers becoming more skilled in breaking work down into smaller features (i.e., feature implementation time stays about the same because fewer developers are working on each feature, making developers available to start on new features).
Software product development is a complicated business and a wide variety of different events and processes are likely to have contributed to the patterns of behavior seen in the data. While developers write the software it is customers who report most of the bugs and one of the goals of following an Agile methodology is rapid response to customer feedback (e.g., deciding which features need to be implemented and which left out). Customer information is not present in the dataset.
Are the same processes generating the apparent two phase behavior?
Any pattern of behavior is generated by a set of processes and when a pattern of behavior changes it is worthwhile asking how the processes driving the behavior changed.
Fitting a statistical distribution to a dataset is useful in that many distributions are known to be generated by processes having specified behaviors. Being able to fit the same distribution to both the pre and post 650 day datasets suggests that the phase change seen was not a fundamental change but akin to turning the volume knob of the distribution parameters one way or the other. If the datasets are best fitted by different distributions then the processes generating the two patterns of behavior are potentially very different.
Of the two characteristics plotted the feature implementation time appears to undergo the largest change of behavior and so the distribution of implementation times for the two phases is analysed here.
Moment | Initial 650 days | After 650 days |
---|---|---|
Median
|
3
|
3
|
Mean
|
7.6
|
4.6
|
Variance
|
116.4
|
35.0
|
Skewness
|
3.3
|
4.9
|
Kurtosis
|
19.2
|
30.4
|
A quick look at the data shows that many features are implemented in a single day and only a few take more than a week, one distribution having this pattern of behavior is the power-law. The table above shows that the variance is much larger than the mean and the distribution has a large positive skew, properties shared by the [negative binomial distribution]. The figure below is a plot of the number of features requiring a given number of elapsed working days for their implementation (top first 650 days, all features finished after 650 days), along with two power-law and a negative binomial distribution fit to the data.
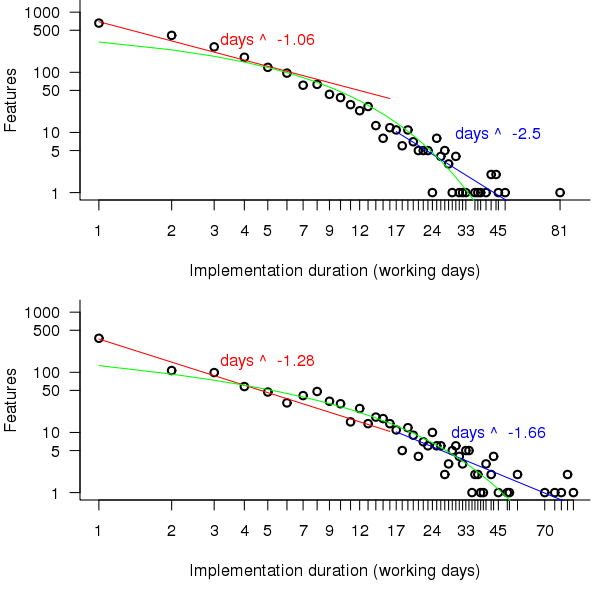
Figure 2. Number of features whose implementation took a given number of elapsed workdays. Top first 650 days, bottom after 650 days. Green line is the fitted negative binomial distribution. Data courtesy of 7digital.
The power-law fits were obtained by splitting the data into two parts, shorter/longer than 16 days (after noticing that visually the combined dataset seemed to have this form, less noticeable in the two subsets) and performing nonlinear regression using nls
to find good fits for the parameters a
and b
(whose initial starting values converged without needing manual tuning).
pow_equ=nls(num.features ~ a*days^b, start=list(a=1200, b =-2)) y=predict(pow_equ, days) lines(days, y) |
While the power-law fits are not very good overall one of them does provide an easy to remember seat of the pants method for approximating the probability of a project taking a small number of days to complete (e.g., for it is
[sum(1/(1:16))
is 3.38]). The approximation is also a reasonable fit for subsets of the features (e.g., different kinds of bugs).
The R package fitdistrplus
contains functions for matching and fitting a dataset against known commonly occurring distribution. The Cullen and Frey graph produced by a call to descdist
suggests that a negative binomial distribution is the best fitting of those tested (agreeing with the ad-hoc conclusion jumped to above).
descdist(p\$Cycle.Time, discrete=TRUE, boot=100) |
The function fitdist
returns values for the parameters providing the appropriate fit to the specified dataset and distribution.
fd=fitdist(p\$Cycle.Time, "nbinom", method="mle") # Fit to a negative binomial distribution size.ct=fd\$estimate[1] mu.ct=fd\$estimate[2] # Plot distribution using fitted parameters plot(dnbinom(1:93, size=size.ct, mu=mu.ct)*length(p\$Cycle.Time), xlim=c(1,90), ylim=c(1,1200), log="xy") |
The figure above shows that the negative binomial distribution could be a reasonable fit if the percentage of single day features was not so high. Two possibilities spring to mind:
- the data does not include any counts for zero days which is one of the possible values supported by the negative binomial distribution (obviously feature implementations cannot take zero days),
- measurement quantization introduces significant uncertainty for shorter implementations, if the minimum unit of measurement were less than 1 day the fit might be much better because some feature implementations take half-a-day while others take a whole day.
It is possible to adjust the negative binomial equation to move the lower bound from zero to one. The package gamlss
supports what is known as zero-truncation and the figure below shows the zero-truncated negative binomial distribution fitted to the pre/post 650 day counts.
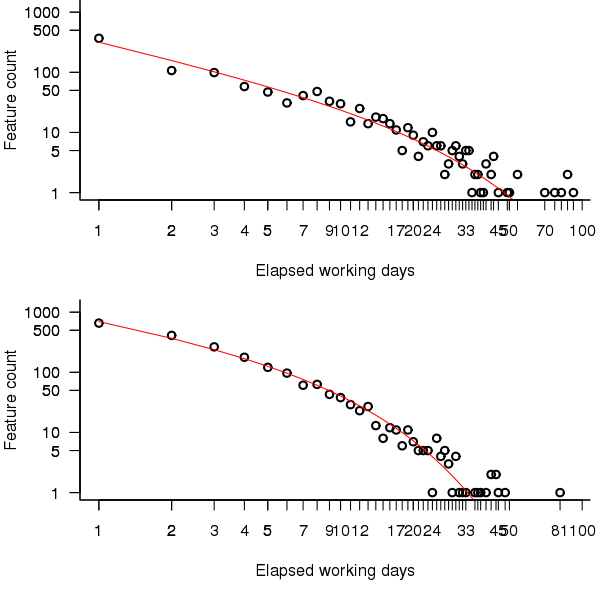
Figure 3. A zero-truncated negative binomial distribution fitted to the number of features whose implementation took a given number of elapsed workdays; top first 650 days, bottom after 650 days. Data courtesy of 7digital.
The quality of fit is much better for the pre 650 day data compared to the post 650 data.
> qual.pre650 AIC log.likelihood 6109.225 -3052.612 > qual.post650 AIC log.likelihood 9923.509 -4959.754 |
Modifying the negative binomial distribution to handle a dataset not containing zeroes improves the fit, can the fit be further improved by adjusting for measurement quantization?
One possibility is to simulate measuring feature implementation in units smaller than a day; the following code multiplies the implementation time by two and randomly decides whether to subtract one, i.e., maps measurements made in days to a possible set of measurements made in half days.
num.features=length(cycle.time) dither=as.integer(runif(num.features, 0, 1) > 0.33) return(2*cycle.time-dither) |
Fitting 1,000 randomly modified half-day measurements and averaging over all results shows that the fit is slightly worse than the original data (as measured by various goodness of fit criteria):
> fit.quality(p\$Cycle.Time[Done.day < 650]) loglikelihood AIC BIC -3438.284 6880.567 6890.575 > rowMeans(replicate(1000, fit.quality(sub.divide(p\$Cycle.Time[Done.day < 650])))) loglikelihood AIC BIC -4072.721 8149.442 8159.450 |
As discussed in the section on [properties of distributions] the negative binomial distribution can be generated by a mixture of [Poisson distribution]s whose means have a [Gamma distribution]. There are other distributions that can be generated through a mixture of Poisson distributions, are any of them a better fit of the data? The Delaporte distribution <book ???> sometimes fits very slightly better and sometimes slightly worse (see chapter source code for details); the difference is not large enough to warrant switching from a relatively well known distribution to one that is rarely covered in text books or supported in software; if data from other projects is best fitted by a Delaporte distribution then a switch may well be worthwhile.
The data subset corresponding to p$Type == "Production Bug"
fits significantly better than the complete dataset (i.e., AIC = 3729) while the fit for the subset p$Type == "MMF"
is comparable to the complete dataset (i.e., AIC of 7251).
Both datsets appear to follow the same distribution, the negative binomial distribution (with zero-truncation), with the initial 650 days having a greater mean and variance than post 650 days. The Poisson distribution is often encountered in processes involving events in time and one can imagine it applying to the various processes involved in the implementation of a feature; why the means of these Poisson distributions might follow a Gamma distribution is harder to fathom and is left for another day (it implies that both the Poisson means are decreasing and that the variance of the means is decreasing)
Do any other equations fit the data? Given enough optional parameters it is always possible to find an equation that is a good fit to the data. The following call to nls
shows that the equation fits the complete dataset rather well.
exp_mod=nls(num.features ~ a*exp(b*days^c), start=list(a=10000, b=-2.0, c=0.4)) |
This equation is unappealing because of its lack of similarity with equations seen in many other areas of research, an exponential whose exponent has the form of raised to a fractional power is rarely encountered. There is a great deal of uncertainty when analysing data for the first time and being able to fit a form of equation used by other researchers provides a big comfort factor.
How many new feature implementations are started on each day?
The table below give the probability of a given number of new feature implementations starting on any day. There are sufficient multi-day implementations that on almost 20% of days no new feature implementations are started. An exponential equation is the commonly encountered form that provides an approximate fit to these values (i.e., ).
0 | 1 | 2 | 3 | 4 | 5 | 6 | 7 | 8 | 9 |
---|---|---|---|---|---|---|---|---|---|
0.18
|
0.12
|
0.15
|
0.1
|
0.099
|
0.081
|
0.076
|
0.043
|
0.033
|
0.029
|
Time dependent patterns in the data
7digital is a growing company and we would expect that the rate of creation of features would increase over time, also as the size of the code base and the customer base increases the rate at which bugs are accepted for fixing is likely to increase.
The number of features developments started per day is one way of comparing different types of features. Plotting this information (see top left) shows that there is a great deal of variation over very short periods of time. This variation can be smoothed using a [rolling mean] to bring out the trends (the rollmean
function in package zoo
); the other plots show 20, 50 and 120 day rolling means for bugs (red) and non-bugs (blue) and the non-bug/bug feature ratio (black).
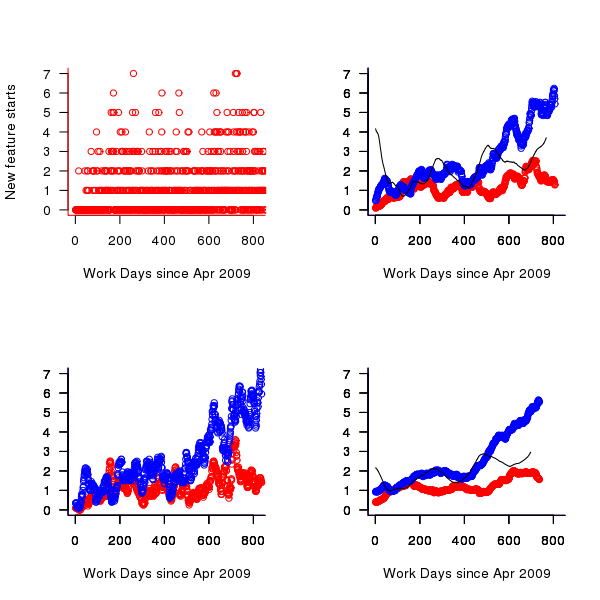
Figure 4. Number of feature developments started on a given work day (red bug fixes, blue non-bug work, black ratio of two values; 20 day rolling mean bottom left, 50 day top right, 120 day bottom right).
Both the number of bugs and non-bug features has trended upwards, as has the ratio between them. While it is tempting to suggest that this increase has been generated by the significant increase in number of developers over the time period, it is also possible the group has become better at dividing work into smaller feature work items or that having implemented the basic core of the products less work is now needed to create new features. The information present in the data is not sufficient to attempt to provide believable explanations for the upward trend.
Time series analysis
A preliminary data analysis technique for time data is to plot the current values against their lagged values for various lags. The output from the R function lag.plot
for the number of in-progress features is shown below; apart from clustering the plots do not show any noticeable relationships in the data.
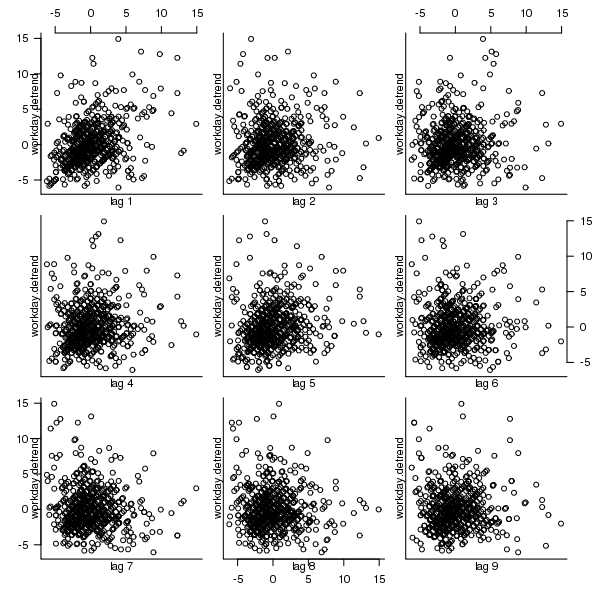
Figure 5. Scatterplot of number of features currently in-progress against various time lags (in working days).
Over longer timescales do the number of in-progress feature implementations have noticeable seasonal variations (e.g., greater in summer and Christmas/year year when developers are likely to be away)?
[Autocorrelation] is the cross-correlation of a time varying signal with itself, i.e., the correlation between a measurement occurring at time and another one occurring at time
; changes in correlation as
increases can be used to infer information about periodic changes over time.
The number of in-progress features appears to be increasing over time (top left of figure below) and this trend away from zero needs to be adjusted for before an autocorrelation is calculated. The feature implementation recording process did not happen over night and took a while before it covered all work performed; comparing a linear fit of all data (pink line of top left of figure below) and all data from January 2010 (red line) shows that this startup period does not significantly bias the growth trend. However, it is possible that patterns of behavior present in the total set of work items over a period are not reflected in the first 250 days of recording (roughly 180 working days) and so these are excluded from this particular analysis. From feature duration measurements we know that over 70% of features take longer than a day to implement, so the data contains a lot of serial dependence which may affect the accuracy of the results.
trend=lm(day.totals ~ time(day.totals)) plot(day.totals, xlab="Days since Apr 2009", ylab="Features in-progress") abline(trend) day.detrend=day.totals - predict(trend) # Subtract out any global trend |
The bottom left of the figure below shows the variation of in-progress features about the trend line. The top right shows the autocorrelation function for this plot, the regular spikes are caused by weekends (when no work took place). Removing weekends from the analysis results in the autocorrelation shown in the bottom right.
Apart from some correlation having a one day lag the autocorrelation drops to zero almost immediately followed by what appear to be small random spikes. These small spikes do not look important enough to follow up. A very similar pattern is seen in the autocorrelation of the two 650-day phases (the initial 650 days has a larger correlation for lags of 2-5 days). It is possible that a seasonal oscillation in feature work exists but is not seen because the data is so noisy (i.e., contains significant variation between adjacent days).
Summing daily values to create weekly totals, which of provides some smoothing, and performing the above analysis again produces essentially the same results.
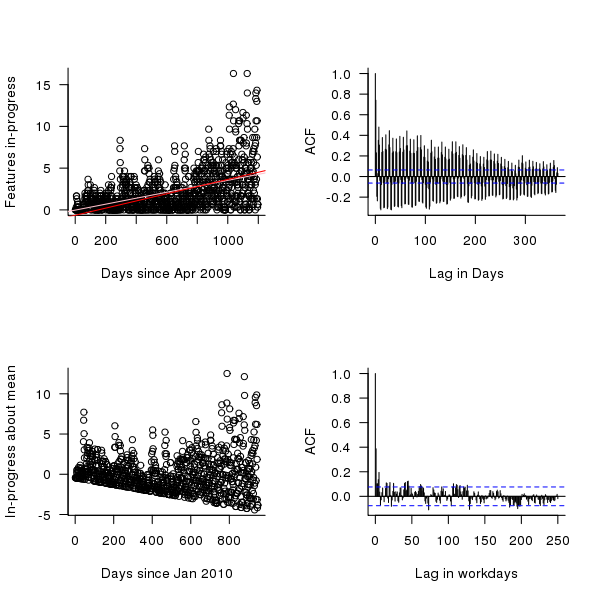
Figure 6. The number of features currently in-production on a given day since April 2009 (top left, pink line is a linear fit of all data, red line a linear fit of the data after day 250), the variation in this number about a linear trend line, excluding the first 250 days (bottom left), the autocorrelation function (top right) and the autocorrelation function with weekends removed from the data (bottom right).
Do reported bugs correlate with new feature releases?
When a feature is released the probability of a new bug being reported increases. Whether different bug probabilities should be assigned to bugfix releases and non-bugfix releases is discussed below. Based on this expectation we would expect to see a [cross correlation] between releases and number of bugs accepted for fixing. The more code a feature contains the more likely it is to contain a bug; however, no information on feature code size is provided so number of implementation work days is used as a measure of feature size.
The data does not specify which bugs belong to which features. It is to be expected that over time the probability of a bug being reported against a feature will decrease, reasons for this behavior include bugfixing, customers no longer using a feature and features being superseded by newer ones.
The figure below is the cross correlation between the ‘size’ of all features recorded as Done on a given day and all bugs recorded as Prioritised on a given date; the top plot is for all non-bugfix feature releases while the bottom plot is for all feature releases.
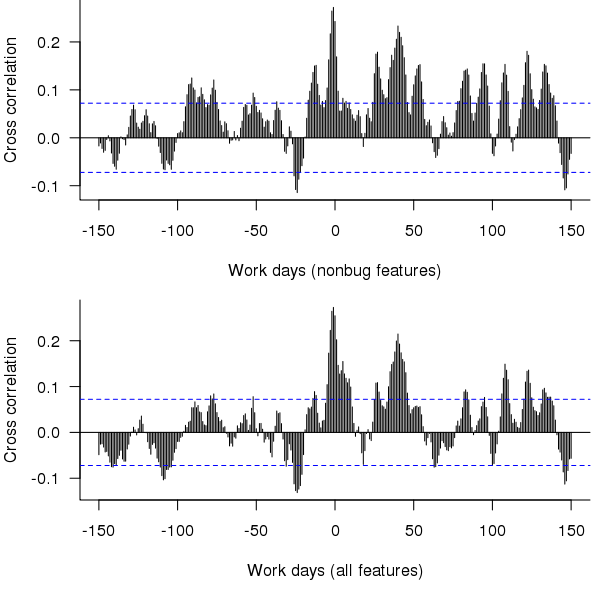
Figure 7. Cross correlation of feature release ‘size’ (top non-bugfix releases, bottom all releases) and date when bugs are prioritised.
The feature/bug cross correlation in the figure above should be zero for negative lags (i.e., no bugs can be reported for features that have not yet been released). One way of interpreting the pattern of correlation is that there some bugs are reported immediately after the release (perhaps by early adopters) followed by more bugs some 20 to 50 working days after release; other interpretations include there being a small amount of signal just visible behind lots of noise in the data or that the approximation used to estimate feature size is too crude.
Using weekly totals produces essentially the same result.
Summary of findings
The distribution of feature implementation times appears to follow a negative binomial distribution (with zero-truncation), with the values for the initial 650 days having a greater mean and variability (i.e., variance) than the following days.
There appears to be too much noise in the data for any time series signal involving mean values or a relationship between releases and bugs to be reliably extracted.
Acknowledgements
Thanks to 7digital for making the data available and being willing to make it public and to Rob Bowley for helping me to understand 7digital’s development environment.
My no loops in R hair shirt
Being professionally involved with analyzing source code, I get to work with a much larger number of programming languages than most people. There is a huge difference between knowing the intricate details of the semantics of a language and being able to fluently program in a language like a native developer. There are languages whose semantics I probably know better than nearly all its users and yet can only code in like a novice, and there are languages whose reference manual I might have read once and yet can write fluently.
I try not to learn new languages in which to write programs, they just clutter my brain. It can be very embarrassing having somebody sitting next to me while I write an example and not be able to remember whether the language I am using requires a then
in its if-statement or getting the details of a print statement wrong; I am supposed to be a computer language expert.
Having decided to migrate from being a casual R user to being a native user (my current status is somebody who owns more than 10 books that make extensive use of R) I resolved to invest the extra time needed to learn how to write code the ‘R-way’ (eighteen months later I’m not sure that there is an ‘R way’ in the sense that could be said to exist in other languages, or if there is it is rather diffuse). One of my self-appointed R-way rules is that any operation involving every element of a vector should be performed using whole vector operations (i.e., no looping constructs).
Today I was analyzing the release history of the Linux kernel and wanted to get the list of release dates for the current version of the major branch; I had a list of dates for every release. The problem is that when a major release branch is started previous branches, now in support only mode, may continue to be maintained for some time, for instance after the version 2.3 branch was created the version 2.2 branch continued to have releases made for it for another five years.
The obvious solution to removing non-applicable versions from the release list is to sort on release date and then loop through the elements, removing those whose version number was less than the version appearing before them in the list. In the following excerpt the release of 2.3.0 causes the following 2.2.9 release to be removed from the list, also versions 2.0.37 and 2.2.10 should be removed.
Version Release_date 2.2.8 1999-05-11 2.3.0 1999-05-11 2.2.9 1999-05-13 2.3.1 1999-05-14 2.3.2 1999-05-15 2.3.3 1999-05-17 2.3.4 1999-06-01 2.3.5 1999-06-02 2.3.6 1999-06-10 2.0.37 1999-06-14 2.2.10 1999-06-14 2.3.7 1999-06-21 2.3.8 1999-06-22 |
While this is an easy problem to solve using a loop, what is the R-way of solving it (use the xyz
package would be the answer half said in jest)? My R-way rule did not allow loops, so a-head scratching I did go. On the assumption that the current branch version dates would be intermingled with releases of previous branches I decided to use simple pair-wise comparison (which could be coded up as a whole vector operation); if an element contained a version number that was less than the element before it, then it was removed.
Here is the code (treat step
parameter was introduced later as part of the second phase tidy up; data here):
ld=read.csv("/usr1/rbook/examples/regression/Linux-days.csv") ld$Release_date=as.POSIXct(ld$Release_date, format="%d-%b-%Y") ld.ordered=ld[order(ld$Release_date), ] strip.support.v=function(version.date, step) { # Strip off the least significant value of the Version id v = substr(version.date$Version, 1, 3) # Build a vector of TRUE/FALSE indicating ordering of element pairs q = c(rep(TRUE, step), v[1:(length(v)-step)] <= v[(1+step):length(v)]) # Only return TRUE entries return (version.date[q, ]) } h1=strip.support.v(ld.ordered, 1) |
This pair-wise approach only partially handles the following sequence (2.2.10 is greater than 2.0.37 and so would not be removed).
2.3.6 1999-06-10 2.0.37 1999-06-14 2.2.10 1999-06-14 2.3.7 1999-06-21 |
The no loops rule prevented me iterating over calls to strip.support.v
until there were no more changes.
Would a native R speaker assume there would not be many extraneous Version/Release_date pairs and be willing to regard their presence as a minor data pollution problem? If so, I have some way to go before I might be able to behave as a native.
My next line of reasoning was that any contiguous sequence of non-applicable version numbers would probably be a remote island in a sea of applicable values. Instead of comparing an element against its immediate predecessor, it should be compared against an element step
back (I chose a value of 5).
h2=strip.support.v(h1, 5) |
The original vector contained 832 rows, which was reduced to 745 and then down to 734 on the second step.
Are there any non-loop solutions that are capable of handling a higher density of non-applicable values? Do tell if you can think of one.
Update (a couple of days later)
Thanks to Charles Lowe, Wojtek and kaz_yos for their solutions using cummax
, a function that I was previously unaware of. This was a useful reminder that what other languages do in the syntax/semantics R surprisingly often does via a function call (I’m still getting my head around the fact that a switch-statement is implemented via a function in R); as a wannabe native R speaker I need to remove my overly blinked language approach to problems and learn a lot more about the functions that come as part of the base system
Success does not require understanding
I took part in the second Data Science London Hackathon last weekend (also my second hackathon) and it was a very different experience compared to the first hackathon. Once again Carlos and his team really looked after us.
- The data was released 24 hours before the competition started and even though I had spent less than half an hour looking at it, at the start of the competition I did not feel under any time pressure; those 24 hours allowed me to get used to the data and come up with some useful looking ideas.
- The instructions for the first competition had suggested that people form teams of 3-5 and there was a lot of networking happening before the start time. There was no such suggestion this time and as I networked around looking for people to work with I was surprised by the number of people who wanted to work alone; Jonny and Kannappan were the only members from my previous team (the Outliers) who had entered this event, with Kannappan wanting to work alone and Jonny joining me to create a two person team.
- There was less community spirit this time, possible reasons include a lot more single person teams sitting in the corner doing their own thing, fewer people attending (it is the middle of the holiday season), fewer people staying over until the Sunday (perhaps single person teams got disheartened and left or the extra 24 hours of data access meant that teams ran out of ideas/commitment after 36 hours) or me being reduced to a single person team (Jonny had to leave at 20:00) meant I paid more attention to what was happening on the floor.
The problem was to predict what ratings different people would give to various music artists. We were given data involving 50 artists and 48,645 users (artists and users were anonymous) in five files (one contained the training dataset and another the test dataset).
A quick analysis of the data showed that while there were several thousand rows of data per artist there were only half a dozen rows per person, a very sparse dataset.
The most frequent technique I heard mentioned during my initial conversations with attendees was machine learning. In my line of work I am constantly trying to understand what is going on (the purpose of this understanding is to control and make things better) and consider anybody who uses machine learning as being clueless, dim witted or just plain lazy; the problem with machine learning is that it gives answers without explanations (ok decision trees do provide some insights). This insistence on understanding turned out to be my major mistake, the competition metric is based on correctness of answers and not on how well a competitor understands the problem domain. I had a brief conversation with a senior executive from EMI (who supplied the dataset and provided some of the sponsorship money) who showed up on Sunday morning and he had no problem with machine learning providing answers and not explanations.
Having been overly ambitious last time team Outliers went for extreme simplicity and started out with the linear model glm(Rating ~ AGE + GENDER...)
being built for each artist (i.e., 50 models). For a small amount of work we got a score of just over 21 and a place of around 70th on the leader board, now we just needed to include information along the lines of “people who like Artist X also like Artist Y”. Unfortunately the only other member of my team (who did not share my view of machine learning and knew something about it) had a prior appointment and had to leave me consuming lots of cpu time on a wild goose chase that required me to have understanding.
The advantages of being in a team include getting feedback from other members (e.g., why are you wasting your time doing that, look how much better this approach is) and having access to different skill sets (e.g., knowing what magic pixie dust values to use for the optional parameters to machine learning routines). It was Sunday morning before I abandoned the ‘understanding’ approach and started thrashing around using various machine learning techniques, which told me that people demographics (e.g., age and gender) were not particularly good predictors compared to other data but did did not reduce my score to the 13-14 range that could be seen on the leader board’s top 20.
Realizing that seven hours was not enough time to learn how to drive R’s machine learning packages well enough to get me into the top ten, I switched tack and spent a lot more time wandering around chatting to people; those whose score was worse than mine were generally willing to chat. Some had gotten completely bogged down in data cleaning and figuring out how to handle missing data (a subject rarely covered in books but of huge importance in real life), I was surprised to find one team doing most of their coding in SQL (my suggestion to only consider Age+Gender improved their score from 35 to 22), I mocked the people using Clojure (people using a Lisp derived language think they have discovered the one true way and will suffer from self doubt if they are not mocked regularly). Afterwards it struck me that well over 50% of attendees were not British (based on their accents), was this yet another indicator of how far British Universities had dumbed down mathematics teaching that natives did not feel up to the challenge (well done to the Bristol undergraduate who turned up) or were the most gung-ho technical folk in London those who had traveled here to work from abroad?
The London winner was Dell Zhang, the only other person sitting at the table I was on (he sat opposite me throughout the competition), who worked quietly away for the whole 24 hours and seemed permanently unimpressed by the score he was achieving; he described his technique as “brute force random forest using Python (the source will be made available on the Data Science website).
Reading through posts made by competitors after the event was as interesting as last time. Factorization Machines seems to be the hot new technique for making predictions based on very sparse data and the libFM is the software I needed to know about last weekend (no R package providing an interface to this C++ code available yet).
Impact of hardware characteristics on detectable fault behavior
Preface. This is the first of what I hope will be many posts analysing experimental data, that will eventually end up in my empirical software engineering with R book (this experiment was chosen because it happens to be the one I am currently working on; having just switched to using Asciidoc I have a backlog of editing to do on previously written analysis, also I have to figure out a way to fix [bracketed words]).
Don’t worry if you don’t know anything about the statistics used. I am aiming to provide information to meet the needs of two audiences (whether or not I fail on both counts remains to be seen):
- Those who want to some idea of what facts are known about a particular software engineering topic. Hopefully reading the introduction+conclusion will enable these readers to form an opinion about the current state of knowledge (taking my statistical analysis on trust).
- Those who are looking for ideas that can be used to analyse a problem they are trying to solve. Hopefully, somewhere among my many analyses will be something that looks like it could be applied to the reader’s problem and motivates them to go off and learn something about the statistics (if they are not already familiar with it; once written the book will obviously help out here).
Forward. The following analysis produces a negative result, something that happens a lot in experiments in all fields of research. It has been included to illustrate the importance of checking the statistical power of an experiment, i.e., how likely the experiment will detect an effect if one is present; it is very easy to fall into the trap of thinking that because lots of tests were done any effect that exists will be detected.
The authors ran an interesting experiment which as far as I know is the only published empirical analysis of intermittent software faults (please let me know if you are aware of other work) and made some mistakes in their statistical analysis. I have made plenty of mistakes in experiments I have run, some of which have found there way into the published write up. The key attribute of an experimentalist is to learn and move on.
A fault does not always noticeably change the behavior of a program when it is executed, apparently correct program execution can occur in the presence of serious faults.
A study by Syed, Robinson and Williams <book Syed_10> investigated how the number of noticeable failures caused by known faults in Mozilla’s Firefox browser varied with processor speed, system memory, hard disc size and system load. A total of 11 known faults causing intermittent failure were selected and nine different hardware configurations were selected. The conditions required to exhibit each fault were replicated and Firefox was executed 10 times for each of hardware configuration, counting the number of noticeable program failures; the seven faults and nine hardware configurations listed in the table below generated a total of 10*7*9 = 630 different executions (four faults either always or never resulted in an observed failure during the 10 runs).
Data
The following table contains the observed number of failures of Firefox for the given fault number when run on the specified hardware configuration.
Mhz-Mb-Gb | 124750 | 380417 | 410075 | 396863 | 494116 | 264562 | 332330 |
---|---|---|---|---|---|---|---|
667-128-2.5 |
4 |
10 |
6 |
5 |
2 |
3 |
5 |
667-256-10 |
4 |
8 |
8 |
6 |
4 |
3 |
8 |
667-1000-2.5 |
4 |
7 |
3 |
4 |
3 |
1 |
8 |
1000-128-10 |
3 |
10 |
3 |
6 |
0 |
1 |
1 |
1000-256-2.5 |
3 |
9 |
0 |
6 |
0 |
1 |
2 |
1000-1000-10 |
2 |
9 |
4 |
5 |
0 |
0 |
1 |
2000-128-2.5 |
0 |
10 |
5 |
6 |
0 |
0 |
0 |
2000-256-10 |
2 |
8 |
5 |
7 |
0 |
0 |
0 |
2000-1000-10 |
1 |
7 |
3 |
5 |
0 |
0 |
0 |
Predictions made in advance
There is no prior theory suggesting how the selected hardware characteristics might influence the outcome from this experiment. The analysis is based on searching for a pattern in the results and so the significance level needs to be adjusted to take account of the number of possible patterns that could exist (e.g., using the [Bonferroni correction]).
If we simplify the failure counts by labelling them as one of Low/Medium/High, then there are two arrangements of the failure counts (i.e., low/medium/high and high/medium/low) that would result in a strong correlation for cpu_speed, two arrangements for memory and two for disc size; a total of 6 combinations that would result in a strong correlation being found.
The [Bonferroni correction] adjusts the significance level by dividing by the number of tests, in this case 0.05/6 = 0.0083.
If the failure counts occurred in a random order what is the probability of a strong correlation between failure count and one of the hardware attributes being found? Based on the Low/Medium/High labelling scheme there are 9!/(3! 3! 3!) = 1680 combinations of these counts over 9 slots, giving a 1 in 1680/6 = 280 chance of purely random behavior producing a strong correlation.
The experiment investigated the characteristics of 11 faults. If there is a 1 in 280 chance of finding a strong correlation when analyzing one fault there is approximately a 1 in 24 chance of finding at least one strong correlation when analysing 11 different faults.
Response variable
The response variable takes the form of a proportion whose value varies between 0 and 1, the number of failures out of 10 executions.
Applicable techniques
The following techniques might be used to analyse this data:
- [Factorial design]. This is a way of organizing experiment configurations that is designed to extract the most information for the total number of program runs made. It would be inefficient not to use the results from some hardware configurations just because they are not needed in the factorial design and no results are available for some configurations required by a factorial design (or a [Plackett-Burman] design).
-
Fitting the data using a linear model. A standard linear model, created using R’s lm function, would not be appropriate because of the following two problems:
- this kind of model is likely to make predictions that fall outside the range 0 to 1, something that cannot happen for proportional data,
- this approach assumes that the variance is constant across measurements and unless the proportions involved are very close to each other this requirement will not be met ([proportional data] from a [binomial distribution] has variance p(1-p)).
However, a generalised linear model would not suffer from these problems. There are several [link functions] that could be used:
- the Poisson distribution, is widely used for modelling faults but requires that the mean and variance have the same value, a property that does not apply to proportional data.
- the Binomial distribution, can handle data having the characteristics present here.
The proportional data is specified in the call to the glm function by having the response variable contain two columns, one containing the number of failures (that is what is being predicted in this case) and the other the number of non-failures. The code looks something like the following (see complete example and data):
y=cbind(fail_count, 10-fail_count) glm(y ~ cpu_speed+memory+disk_size, data=ff_data, family=binomial) |
In this kind of GLM it is assumed that the [residual deviance] is the same as the [residual degrees of freedom]. If the residual deviance is greater than the residual degrees of freedom then [overdispersion] has occurred, which happens for fault 380417. To handle overdispersion the family needs to be changed from binomial to quasibinomial, which in the case of fault 380417 changes the p-value of the fit from 0.0348 to 0.0749.
The analysis of each fault finds that only one of them, 332330, has a significance level within the specified acceptable bounds; this has a negative correlation with CPU speed (i.e., observed failures decrease with clock speed).
With only one faults found to have any significant hardware configuration effects we have to ask about the probability of this experiment finding an effect if one was present.
An analysis of the [statistical power] of an experiment investigating the difference between proportions for two hardware configurations (i.e., the percentage of observed failures) needs to know the value of those proportions, the number of runs (10 in this case) and the desired p-value (0.05); to simplify things the plot below is based on using the value of the lowest proportion and the difference between it and the higher proportion. The left plot shows the power achieved (y-axis) there does exist a given difference in proportions (x-axis), the three lowest proportions of 0.05, 0.25 and 0.5 are shown (the result is symmetric about 0.5 and so the plot for 0.75 and 0.95 would be the same as 0.25 and 0.05 respectively), and where there were 10 and 50 runs involving the same fault case.
It can be seen that unless a change in the hardware configuration causes a large change in the number of visible failures then the chance of a difference being detected in results from 10 runs is well below 0.5 (i.e., less than a 50% chance of detecting a difference at a p-value of 0.05 or better).
The right plot in the figure gives the number of runs that need to be made to have a 80% chance of detecting, between two different hardware configurations, the difference in proportion listed on the x-axis, at a significance of 0.05.
It can be seen that if hardware charactersitics account for only 10% of the difference in failure rate over 100 runs would be needed to detect it.
Conclusion
Faults in Firefox that caused intermittent failures were investigated looking for a correlation with system cpu speed, memory or disc size. One fault showed a strong correlation with cpu speed (there is a 1 in 24 chance that one of the investigated faults would have some kind of strong correlation). This experiment may not have found a significant correlation between observed failure rate and hardware configuration because the number of separate runs for each fault (i.e., 10) had [low power].
Background to my book project “Empirical Software Engineering with R”
This post provides background information that can be referenced by future posts.
For the last 18 months I have been working in fits and starts on a book that has the working title “Empirical Software Engineering with R”. The idea is to provide broad coverage of software engineering issues from an empirical perspective (i.e., the discussion is driven by the analysis of measurements obtained from experiments); R was chosen for the statistical analysis because it is becoming the de-facto language of choice in a wide range of disciplines and lots of existing books provide example analysis using R, so I am going with the crowd.
While my last book took five years to write I had a fixed target, a template to work to and a reasonably firm grasp of the subject. Empirical software engineering has only really just started, the time interval between new and interesting results appearing is quiet short and nobody really knows what statistical techniques are broadly applicable to software engineering problems (while the normal distribution is the mainstay of the social sciences a quick scan of software engineering data finds few occurrences of this distribution).
The book is being driven by the empirical software engineering rather than the statistics, that is I take a topic in software engineering and analyse the results of an experiment investigating that topic, providing pointers to where readers can find out more about the statistical techniques used (once I know which techniques crop up a lot I will write my own general introduction to them).
I’m assuming that readers have a reasonable degree of numeric literacy, are happy dealing with probabilities and have a rough idea about statistical ideas. I’m hoping to come up with a workable check-list that readers can use to figure out what statistical techniques are applicable to their problem; we will see how well this pans out after I have analysed lots of diverse data sets.
Rather than wait a few more years before I can make a complete draft available for review I have decided to switch to making available individual parts as they are written, i.e., after writing a draft discussion and analysis of each experiment I will published it on this blog (along with the raw data and R code used in the analyse). My reasons for doing this are:
- Reader feedback (I hope I get some) will help me get a better understanding of what people are after from a book covering empirical software engineering from a statistical analysis of data perspective.
- Suggestions for topics to cover. I am being very strict and only covering topics for which I have empirical data and can make that data available to readers. So if you want me to cover a topic please point me to some data. I will publish a list of important topics for which I currently don’t have any data, hopefully somebody will point me at the data that can be used.
- Posting here will help me stay focused on getting this thing done.
Links to book related posts
Distribution of uptimes for high-performance computing systems
Break even ratios for development investment decisions
Agreement between code readability ratings given by students
Changes in optimization performance of gcc over time
Descriptive statistics of some Agile feature characteristics
Impact of hardware characteristics on detectable fault behavior
Prioritizing project stakeholders using social network metrics
Preferential attachment applied to frequency of accessing a variable
Amount of end-user usage of code in Firefox
How many ways of programming the same specification?
Ways of obtaining empirical data in software engineering
What is the error rate for published mathematical proofs?
Changes in the API/non-API method call ratio with program size
Honking the horn for go faster memory (over go faster cpus)
How to avoid being a victim of Brooks’ law
Evidence for the benefits of strong typing, where is it?
Hardware variability may be greater than algorithmic improvement
Extracting the original data from a heatmap image
Entropy: Software researchers go to topic when they have no idea what else to talk about
EU rules that computer languages cannot be copyrighted
The European Court of Justice has published its decision in SAS v WPL; the title of the press release says it all “The functionality of a computer program and the programming language cannot be protected by copyright”. To summarise the background, World Programming Ltd developed a system that was capable of emulating the input/output behavior of programs written in what the SAS Institute Inc were claiming to be their copyrighted scripting language, along with various file formats.
According to the Court of Justice, “the Court holds that neither the functionality of a computer program nor the programming language and the format of data files used in a computer program in order to exploit certain of its functions constitute a form of expression. Accordingly, they do not enjoy copyright protection.”
This EU ruling is not quiet what it seems. The SAS v WPL case is before the High Court in London and the EU Court of Justice has been asked for advice based on European Law. So the UK dispute has not yet been decided, but given that the UK is signed up to adhere by EU laws people who know about the legal stuff seem to think the High Court in London will follow the EU ruling. Assuming this, then…
This ruling is not just bad news for SAS, it is also bad news for their competitors. Competition is likely to lead to better/cheaper products for users of the SAS language, resulting in less incentive for them to move to an alternative (the R language included; incidentally what exactly are The R Foundation for Statistical Computing claiming copyright over in that notice that pops up when R is started?)
The Oracle vs. Google Java API lawsuit involves similar territory. There are plenty of details over at Groklaw and I’m not going to go there.
This ruling makes it much more likely that behave-alike implementations of more ‘corporate languages’ will be created, at least in Europe. Previously the threat of a lawsuit would have been enough to deter most people, irrespective of whether what they wanted to do was legal or not.
What languages might we see implemented any time soon? The one that immediately springs to mind for me is Mathematica, which is the leader in its field and a fork of Maxima that supported the Mathematica language would move it out of the ghetto. Octave and Matlab are already very close, so no change there.
I imagine there are corporate languages scattered over every conceivable application domain. A lot of these domains will be sufficiently specialized that there is a very low probability of somebody creating an open source implementation; if it looks like there is money to be made it has become more likely that an alternative commercial implementation will be created.
It looks like being a compiler writer is back as flavor of the month again 🙂
Incompetence borne of excessive cleverness
I have just got back from the 24 hour Data Science Global Hackathon; I was an on-site participant at Hub Westminster in London (thanks to Carlos and his team for doing such a great job looking after us all {around 50 turned up from the 100 who registered; the percentage was similar in other cities around the world}). Participants had to be registered by 11:00 UTC, self form into 3-5 person teams ready for the start at 12:00 UTC and finish 24 hours later. The world-wide event had been organized by our London hosts who told us they expected the winning team to come from those in the room; Team Outliers (Wang, Jonny, Kannappan, Bob, Simon, yours truely and Fran for the afternoon) started in an optimistic mood.
At 12:00 an air-quality training dataset + test points was made available and teams given the opportunity to submit eight predictions in each of the two 12 hour time periods. The on-line submissions were evaluated by Kaggle (one of the sponsors, along with EMC) to produce a mean estimated error that was used to rank teams.
The day before the event I had seen a press release saying that the task would involve air-quality and a quick trawl of the Internet threw up just the R package I needed, OpenAir; I also read a couple of Wikipedia articles on air pollution.
Team Outliers individually spent the first hour becoming familiar with the data and then had a get-together to discuss ideas. Since I had a marker pen, was sitting next to a white-board and was the only person with some gray hair I attempted to manage the herding of the data science cats and later went on to plot the pollution monitor sites on Google Maps as well as producing some visually impressive wind Roses (these did not contribute anything towards producing a better solution but if we had had a client they could have been used to give the impression we were doing something useful).
People had various ideas about the techniques to use for building the best model and how the measurements present in the training set might be used to predict air quality (the training data had names such as target_N_S
, where N
and S
were small integer values denoting the kind of pollution and the site where the measurement was made). The training set included measurements of wind speed/direction data and hours of sunlight, and a couple of members wanted to investigate if these would make good predictors. Team Outliers had people looking at all the fancy stuff you find in textbooks, e.g., ARIMA for time series, svm for machine learning, and I was looking at getting the data into the form needed by OpenAir.
There were all sorts of problems with the data, just like real life, e.g., missing values (lots of them), some kinds of quality (i.e., pollutants) were only measured at one or two sites and fuzzy values such as the ‘most common month’ (what ever that might be). Some people were looking at how best to overcome this data quality problem.
20:30 arrived, and some great food was laid out for dinner, no actual predictions yet, but they would be arriving real soon now. A couple of hours later my data formatting project crashed and burned (being a 32-bit system, R got upset about my request to create a vector needing 5.2 Gig; no chance of using the R-based OpenAir package which needed data in a format different from the one we were given it in). Fifteen minutes to midnight I decided that we either used the eight submissions permitted in the first 12 hours or lost them, and wrote a dozen lines of R that built a linear model using one predictor variable (which I knew from some earlier plots was far from linear, but the coding was trivial, and the lm
function would take no time to build separate models for each of the 30 odd response variables). I submitted the predictions, and we appeared on the score board at number 65 out of 112. Being better than 47 other teams was a bit of a surprise.
Panic over, we realised that the 12 hours ended at 12:00 UTC which was 01:00 BST (British Summer time) and we had another hour. Wang made a couple of submissions that improved our score, and at around 02:00 I went to grab a few hours sleep.
I was back online at 06:00 to find that team Outliers had slipped to 95th place as the Melbourne and San Francisco teams had improved during their daytime. More good food for breakfast at 08:30.
Jonny drew attention to the fact that the mean absolute error in our team’s current score was almost twice as great as that of the sample solution provided with the data. We had long ago dismissed this solution as being too simplistic (it was effectively a database solution in that it calculated the mean value of the various pollutants in the training set at various chunkID
and hour
points which were used as keys for ‘looking up’ prediction values required by the test dataset). Maybe team members ought to focus their attention on tweaking this very simple approach rather than our ‘cleverer’ approaches.
I suggested modifying the sample solution to use the median rather than the mean (less susceptible to outliers), this boosted our ranking back into the 1960s. Jonny and Simon tried using a rolling mean, no improvement; Wang tried other variations, no improvement.
Team Outliers finished the Hackathon in equal 61st, along with 22 other teams, out of 114 submissions.
What did we do wrong? Mistakes include:
- trying to do too much for people with our various skill levels in the time available. For instance, I don’t regularly reformat large data tables or try to calculate the error in machine learning models and while I can easily knock out the code I still have to sit down and think about what needs to be written, something that somebody who does this sort of thing regularly would just know how to do; other team members seemed very familiar with the theory but were not used to churning out code quickly.
- spending too much time studying all the various kinds of measurements available in the training set, many of which were not available in the test dataset. We should have started off ignoring measurements in the training set that were not available in the test set, perhaps looking to using these later if time permitted.
Members of team Outliers enjoyed themselves but were a little crestfallen that our clever stuff was not as good as such a crude, but insightful, approach. Most of us used R, a few made use of awk, Python, spreadsheets and Unix shell.
Our hosts are looking to run more data science hackathons this year, in particular one related to the music industry in a few months time. If you are interested in taking part, keep an eye on their website.
Update (later the next day)
At least one team achieved some good results using ARIMA. Fran had started building an ARIMA model, had to leave and nobody else picked it up; I should have been paying more attention to ensure that ideas did not disappear when people left.
An academic programming language paper about R
The R language has passed another milestone, a paper aimed at the academic programming language community (or at least one section of this community) has been written about it, Evaluating the Design of the R Language by Morandat, Hill, Osvald and Vitek. Hardly earth shattering news, but it may have some impact on how R is viewed by nonusers of the language (the many R users in finance probably don’t care that R seems to have been labeled as the language for doing statistics). The paper is well written and contains some very interesting information as well as a few mistakes, although it will probably read like gobbledygook to anybody not familiar with academic programming language research. What follows has something of the form of an R users guide to reading this paper, plus some commentary.
The paper has roughly three parts, the first gives an overview of R, the second is a formal definition of a subset and the third an initial report of an analysis of R usage. For me and I imagine you dear reader the really interesting stuff is in the third section.
When giving a language overview to people who know other computer languages it makes sense to leverage that knowledge, this is why the discussion has a world view from the perspective of languages rarely associated with R: Scheme, Haskell and CLOS. I found some of the discussion of R constructs to be much more informative and less confusing than that in nearly all R books/tutorials I have read, but then they are written from a detailed operational programming language perspective. One criticism of this overview is that it does not give any hint as to why R has such a large following (saying that users found it more useful than these languages would send the wrong kind of signal ;-).
What is a formal description of a subset of R (i.e., done purely using mathematics) doing in the second part? Well, until recently very little academic software engineering was empirically based and was populated by people I would classify as failed mathematicians without the common sense needed to be engineers. Things are starting to change but research that measures things, particularly people, is still regarded as not being respectable in some quarters. In this case the formal definition is playing the role of a virility symbol showing that the authors are obviously regular guys who happen to be indulging in a bit of empirical research.
A surprising number of papers measuring the usage of real software contain formal definitions of a subset of the language being measured. Subsets are used because handling the complete language is a big project that usually involves one or more people getting a PhD out of the work. The subset chosen have to look plausible to readers who understand the mathematics but not the programming language, broadly handle all the major constructs but not get involved with all the fiddly details that need years of work and many pages to describe.
The third part contains the real research, which is really about one implementation of R and the characteristics of R source in the CRAN and Bioconductor repositories, and contains lots of interesting information. Note: the authors are incorrect to aim nearly all of the criticisms in this subsection at R, these really apply to the current implementation of R and might not apply to a different implementation.
In a previous post I suggested some possibilities for speeding up the execution of R programs that depended on R usage characteristics. The Morandat paper goes a long way towards providing numbers for some of these usage characteristics (e.g., 37% of function parameters are assigned to and 36% of vectors contain a single value).
What do we learn from this first batch of measurements? R users rarely use many of the more complicated features (e.g., object oriented constructs {and this paper has been accepted at the European Conference on Object-Oriented Programming}), a result usually seen for other languages. I was a bit surprised that R programs were only 40% smaller than equivalent C programs. I think part of the reason is that some of the problems used for benchmarking are not the kind that would usually be solved using R and I did not see any ‘typical’ R programs being coded up in C for comparison, another possibility is that the authors were not thinking in R when writing the code.
One big measurement topic the authors missed is comparing their general findings with usage measurements of other languages. I think they will find lots of similar patterns of usage.
The complaint that R has been defined by the successive releases of its only implementation, rather than a written specification, applies to all widely used languages, at least in their early days. Back in the day a major reason for creating language standards for Pascal and then C was so that other implementations could be created; the handful of major languages whose specification was written before the first implementation (e.g., PL/1, Ada) have/are dieing out. Are multiple implementations needed in an Open Source world? The answer seems to be no for Perl and yes for PHP, Ruby etc. The effort needed to create a written specification for the R language might be better invested improving the efficiency of the current implementation so that a better alternative is not needed.
Needless to say the authors suggested committing the fatal programming language research mistake.
The authors have created an interesting set of tools for static and dynamic analysis of R and I look forward to reading more about their findings in future papers.
Parsing R code: Freedom of expression is not always a good idea
With my growing interest in R it was inevitable that I would end up writing a parser for it. The fact that the language is relatively small (the add-on packages do the serious work) hastened the event because it did not look like much work; famous last words. I knew about R’s design and implementation being strongly influenced by the world view of functional programming and this should have set alarm bells ringing; this community have a history of willfully ignoring some of the undesirable consequences of their penchant of taking simple ideas and over generalizing them (i.e., I should have expected hidden complications).
While the official R language definition only contains a tiny fraction of the information needed to create a full implementation I decided to use it rather than ‘cheat’ and look at the R project implementation sources. I took as my oracle of correctness the source code of the substantial amount of R in its 3,000+ package library. This approach would help me uncover some of the incorrect preconceived ideas I have about how R source fits together.
I started with a C lexer and chopped and changed (it is difficult to do decent error recovery in automatically generated lexers and I prefer to avoid them). A few surprises cropped up **
is supported as an undocumented form of ^
and by default ]]
must be treated as two tokens (e.g., two ]
in a[b[c]]
but one ]]
in d[[e]]
, an exception to the very commonly used maximal munch rule).
The R grammar is all about expressions with some statement bits and pieces thrown in. R operator precedence follows that of Fortran, except the precedence of unary plus/minus has been increased to be above multiply/divide (instead of below). Easy peasy, cut and paste an existing expression grammar and done by tea time :-). Several tea times later I have a grammar that parses all of the R packages (apart from 80+ real syntax errors contained therein and a hand full of kinky operator combinations I’m not willing to contort the grammar to support). So what happened?
Two factors accounts for most of the difference between my estimate of the work required and the actual work required:
- my belief that a well written grammar has no ambiguities (while zero is a common goal for many projects a handful might be acceptable if the building is on fire and I have to leave). A major advantage of automatic generation of parser tables from a grammar specification is being warned about ambiguities in the grammar (either shift/reduce or reduce/reduce conflicts). At an early stage I was pulling my hair out over having 59 conflicts and decided to relent and look at the R project source and was amazed to find their grammar has 81 ambiguities!
I have managed to get the number of ambiguities down to the mid-30s, not good at all but it will have to do for the time being.
- some of my preconceptions about of how R syntax worked were seriously wrong. In some cases I spotted my mistake quickly because I recognized the behavior from other languages I know, other misconceptions took a lot longer to understand and handle because I did not believe anybody would design expression evaluation to work that way.
The root cause of the difference can more concretely be traced to the approach to specifying language syntax. The R project grammar is written using the form commonly seen in functional language implementations and introductory compiler books. This form has the advantage of being very short and apparently simple; the following is a cut down example in a form of BNF used by many parser generators:
expr : expr op expr | IDENTIFIER ; op : &' | '==' | '>' | '+' | '-' | '*' | '/' | '^' ; |
This specifies a sequence of IDENTIFIER
s separated by binary operators and is ambiguous when the expression contains more than two operators, e.g., a + b * c
can be parsed in more than one way. Parser generators such as Yacc will complain and flag any ambiguity and pick one of the possibilities as the default behavior for handling a given ambiguity; developers can specify additional grammar information in the file read by Yacc to guide its behavior when deciding how to resolve specific ambiguities. For instance, the relative precedence of operators can be specified and this information would be used by Yacc to decide that the ambiguous expression a + b * c
should be parsed as-if it had been written like a + (b * c)
rather than like (a + b) * c
. The R project grammar is short, highly ambiguous and relies on the information contained in the explicitly specified relative operator precedence and associativity directives to resolve the ambiguities.
An alternative method of specifying the grammar is to have a separate list of grammar rules for each level of precedence (I always use this approach). In this approach there is no ambiguity, the precedence and associativity are implicitly specified by how the grammar is written. Obviously this approach creates much longer grammars, there will be at least two rules for every precedence level (19 in R, many with multiple operators). The following is a cut down example containing just multiple, divide, add and subtract:
... multiplicative_expression: cast_expression | multiplicative_expression '*' cast_expression | multiplicative_expression '/' cast_expression ; additive_expression: multiplicative_expression | additive_expression '+' multiplicative_expression | additive_expression '-' multiplicative_expression ; ... |
The advantages of this approach are that, because there are no ambiguities, the developer can see exactly how the grammar behaves and if an ambiguity is accidentally introduced when editing the source it should be noticed when the parser generator reports a problem where previously there were none (rather than the new ambiguity being hidden in the barrage of existing ones that are ignored because they are so numerous).
My first big misconception about R syntax was to think that R had statements, it doesn’t. What other languages would treat as statements R always treats as expressions. The if
, for
and while
constructs have values (e.g., 2*(if (x == y) 2 else 4)
). No problem, I used Algol 68 as an undergraduate, which supports this kind of usage. I assumed that when an if
appeared as an operand in an expression it would have to be bracketed using ()
or {}
to avoid creating a substantial number of parsing ambiguities; WRONG. No brackets need be specified, the R expression if (x == y) 2 else 4+1
is ambiguous (it could be treated as-if it had been written if (x == y) 2 else (4+1)
or (if (x == y) 2 else 4)+1
) and the R project grammar relies on its precedence specification to resolve the conflict (in favor of the first possibility listed above).
My next big surprise came from the handling of unary operators. Most modern languages give all unary operators the same precedence, generally higher than any binary operator. Following Fortran the R unary operators have a variety of different precedence levels; however R did not adopt the restrictions Fortran places on where unary operators can occur.
I assumed that R had adopted the restrictions used by other languages containing unary operators at different precedence levels, e.g., not allowing a unary operator token to follow a binary operator token (i.e., there has to be an intervening opening parenthesis); WRONG. R allows me to write 1 == !1 < 0
, while Fortran (and Ada, etc) require that a parenthesis be inserted between the operands == !
(hopefully resulting in the intent being clearer).
I had to think a bit to come up with an explicit set of grammar rules to handle R unary operator's freedom of expression (without creating any ambiguities).
Stepping back from the details. My view is that programming language syntax should be designed to reduce the number of mistakes developers make. Requiring that brackets appear in certain contexts helps prevent mistakes by the original author and subsequent readers of the code.
Claims that R (or any other language) syntax is 'natural' is clearly spurious and really no more than a statement of preference by the speaker. Our DNA has not yet been found to equip us to handle one programming language better than another.
Over the coming months I hope to have the time to analyse R source looking for faults that might not have occurred had brackets been used. Also how much code might be broken if R started to require brackets in certain contexts?
An example of the difference that brackets can make is provided by the handling of the unary !
operator in R and C/C++/Java/etc. Take the expression !x > y
, which R parses as-if written !(x > y)
and C/C++/Java/etc as if written (!x) > y
. I would not claim that either is better than the other from the point of view of developers getting the behavior right, I know that some C programmers get it wrong and I suspect that some R programmers do too.
By increasing the precedence of unary plus/minus the R designers ensured that 8/-2/2
was parsed like (8/-2)/2
rather than 8/(-2/2)
.
Recent Comments